Digital measurement & attribution
Digital attribution is the measurement of the contribution of digital touchpoints such as search, display and video on demand (VOD) in driving digital call to actions including sales, customer acquisitions and quotes. Third party cookies played a crucial role in the tracking of the digital behaviour of consumers, building information based on the interactions (clicks / impressions) with a brand’s digital marketing prior to conversion.
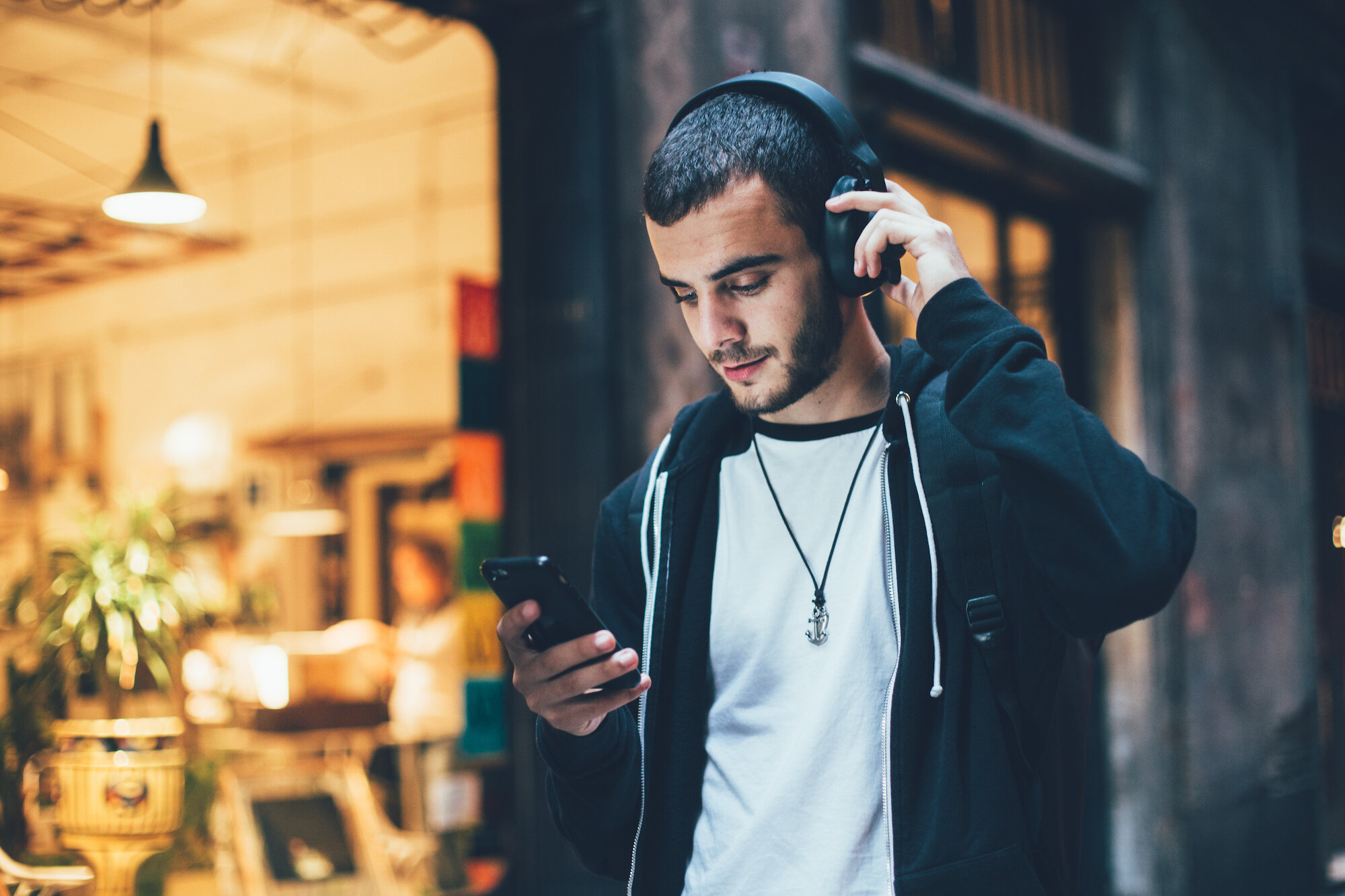
Multi Touch Attribution
Multi Touch Attribution (MTA) helps to understand the contribution of each digital channel and is used for day-to-day management of online advertising and ad tactics. Its central goal is to “adapt performance”. It helps to answer following questions:
- Which campaigns work best?
- Which creatives work best?
- Which advertised products work best?
- Which tactics work best?
- Which sites/publishers work best?
The final objective is to optimise the tactics to get a better return of the digital investment.
The fact that third party cookies are rendered completely obsolete, marks one of the most significant changers in the history of digital media. The gradual depreciation required a pro-active mindset in reinventing Multi Touch Attribution. The last years two approaches popped-up, revitalizing MTA. Both are 3rd party cookie independent and thus future proof.
The solutions
1.
The first solution is to use Marketing Mix Modelling techniques on daily data (see the next section about MMM). The most performing techniques of modern MMM are used to get the most out of the data. Granularity is increased, allowing us to work with more recent data, for example 3 months, instead of 3 years. Sometimes extra levels of granularity are added by including geographic layers or even stores, applying random effects.
2.
The second solution is data driven attribution based on synthetic data. Customer journeys are recreated based on a "pairing" technique, combining data from different platforms in a smart way. A vast majority of the "pairing" can be done deterministically if you get the set-up right as a marketeer. A data-driven attribution method based on recurrent neural network AI solution is then applied, considering both the order of platforms and the quality of site visits. Linking it to the CRM permits to understand conversion paths for first time buyers compared to repeat buyers for example. This process is run overnight and provides daily fresh data to optimise.
Marketing Mix Modelling
Marketing Mix Modelling (MMM) is a method to determine the impact of all factors that can influence your sales results. MMM doesn’t only take marketing expenditure or media deployment into consideration but examines other variables such as external economic influences and changing market conditions. The result is a deeper understanding of the effectiveness of marketing strategies and the ROI of marketing investments. MMM has been used since the 1960’s but is experiencing something of a revival today. It will continue to grow in importance due to the following:
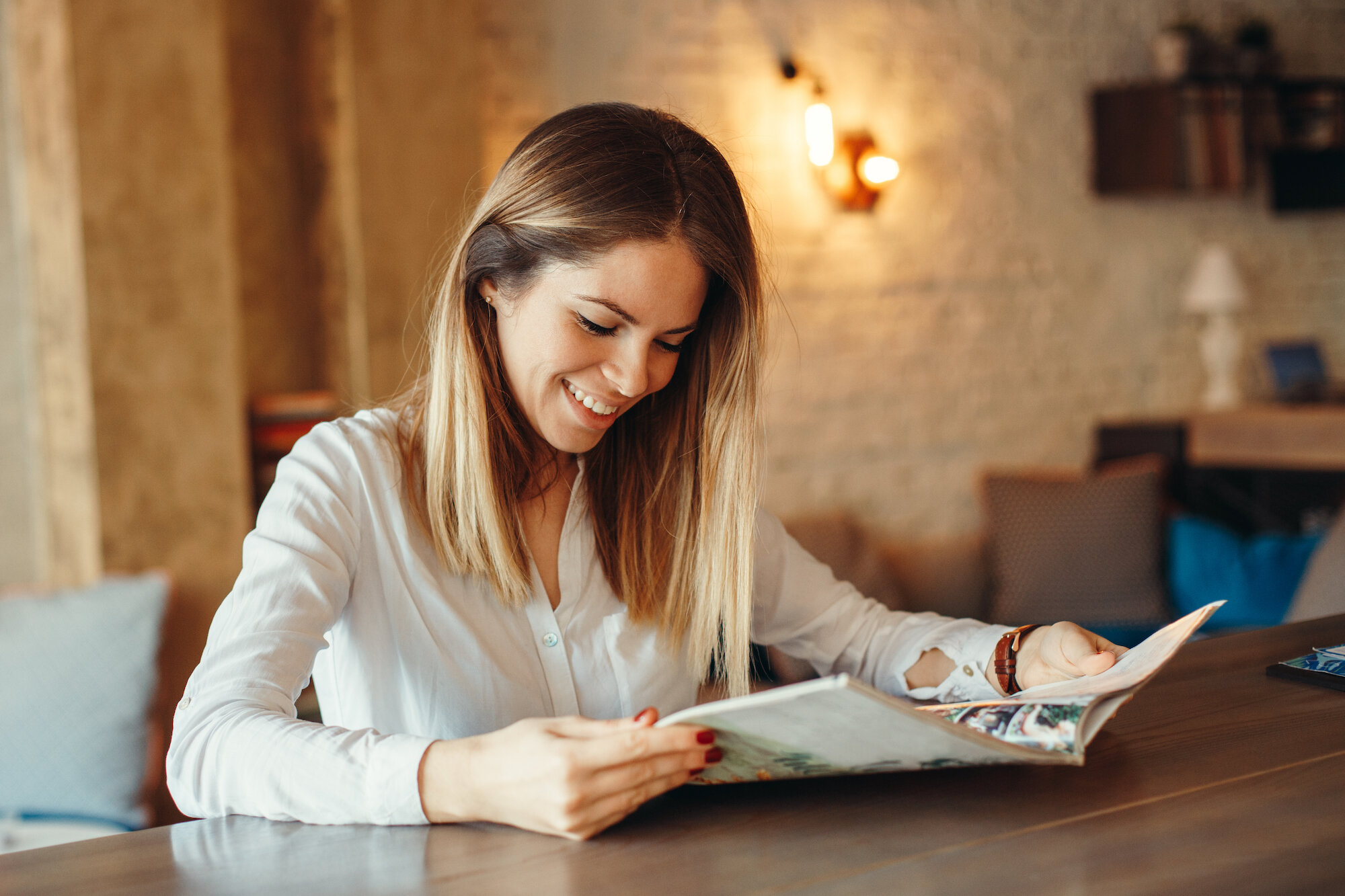
1.
Maximising ROI through data-driven decision-making
In an increasingly competitive market, it’s important to spend your marketing budget as efficiently as possible. Marketeers need to justify their decisions and investments by using hard, measurable data.
2.
The end of the cookie era due to privacy restrictions
Cookies, once the cornerstone of online tracking and targeting, are rapidly losing their value due to privacy restrictions. Working only with aggregated data, MMM does not suffer from the 'last click' attribution error and is the solution for measuring and analysing marketing effectiveness.
3.
SaaS via Facebook and tech vendors
Building on innovations such as cloud computing, online dashboarding and via investments in statistical knowledge, MMM is being repackaged as a user-friendly Software As a Service (SaaS) solution from mainly Facebook and a longtail of tech vendors (Pecan AI, Northbeam,...). The most distinguishing feature of SaaS MMM is that it costs less, is faster and requires fewer resources than traditional MMM.
Even though MMM offers us valuable insights, there are plenty of challenges
Marketing Mix Modelling is often portrayed as a fast, simple method to answer difficult questions, but nothing could be further from the truth. MMM is a demanding project that requires clear ownership and requires a lot of coordination between business and marketing teams, media experts and data scientists. MMM has a minimum lead time of one month.
One of the main bottlenecks is the quality and consistency of gathered data. It’s essential that the data is comprehensive (at least one year), complete (ideally as many variables as possible), accurate and centrally maintained and reported.
No single model or method gives the best outcome. There are many different types of models, each one has its own advantages and disadvantages, which can be applied to the data to a greater or lesser extent. As a result, a SaaS approach is rarely the best solution. A data scientist or external partner is needed for statistical analysis, model selection and evaluation.
In closing, here are some key learnings about media & MMM:
1.
Traditional MMM models focus on the immediate and often short-term impact of media touchpoints. But understanding the long-term effects of media and the brand's impact on sales requires additional data and models. Some studies indicate that about 50% of media's impact comes from the long-term.
2.
MMM models used for media mix optimisation have a clear preference for media with continuous time series (such as TV) versus more ad hoc media (such as daily or monthly magazines). Bias is also possible when comparing broad reach media with regional media or highly targeted touchpoints.
3.
MMM is not a holy grail to boost sales! The unpredictable is impossible to predict and it is more a way to validate and optimise investments in marketing touchpoints rather than a way to predict sales.
Step 1
Defining objectives:
A successful MMM analysis starts with measurable objectives. What do you want to achieve with your marketing efforts? Do you want to increase sales, raise brand awareness or promote customer loyalty?
Step 2
Data collection:
Collecting accurate and comprehensive data is crucial for success. This is more easily said than done. A vast amount of data is required: marketing spend, different marketing channels, sales performance, competition and other relevant factors are just a few key data that need to be collected.
Step 3
Data preprocessing:
Before data can be analysed, it must be prepared and processed. This includes cleaning the data to correct any inaccuracies or inconsistencies.
Step 4
Building the model:
In this phase, analysts develop statistical models that can capture the relationship between marketing efforts and sales performance. These models can include various techniques, such as linear regression, time series analysis or machine learning algorithms. The most commonly used models by DPG include classical linear regression with or without ridge variant, Bayesian linear model, non-linear models Random forest & XGBoost and Structural Equation Models (SEM).
Step 5
Model validation:
The model is tested and validated to ensure that it is accurate and reliable. This includes comparing the model's predicted results with historical data to assess accuracy.
Step 6
Scenario analysis:
Once validated, the model can be used to analyse different scenarios and answer "what if" questions. For example, marketeers can test how changes in the marketing budget or shifts between channels might affect sales results.
Step 7
Implementation and optimisation:
The insights gained are used to adapt and optimise marketing strategies. Marketing simulations can be used to evaluate the impact of changes before they are implemented.
Step 8
Monitoring and adjustment:
MMM can be a continuous process. After implementation, marketeers continue to monitor and adjust the performance of their marketing mix based on new data and changing circumstances.